Small data is the new big data.
Today’s approach to data science problems is focused on big data & deep learning. This combination of abundant data and very high-dimensional models has brought about highly effective solutions in applications such as image- and voice-recognition or -classification, as well as text- and image-generation. However, in many real-world use cases – in business, industry, or research – data is often not as abundant as one initially assumes, because a large portion of the data may not be applicable to the problem at hand, because there has been a sudden regime-switch recently, or because the statistical properties of the data have slowly shifted over time. In some cases, only a tiny fraction of the data points that are initially deemed to be outliers or artifacts actually contain most of the signal.
Regime switches, temporal heterogeneity, and critical outliers (“black swans”) are the tell-tale signs of complex systems, i.e. systems that are made from many interconnected parts that interact in non-linear manners. Complex systems are everywhere: the cells in our body interact to make up organs, different traders interact to make up (more or less) functioning financial markets, the atmospheric layers make up our climate system, the lobbyists and government agencies make up the set of policies that govern our daily life. Due to the non-linear interactions of its parts, the system as a whole then shows emergent features that cannot be easily derived from looking at the parts: our livers filter toxins from blood, atmospheric layers create hurricanes, and so on.
It is thus to no surprise that in finance, policy-making, or medicine, valuable data is often a scarce resource and that the signals one searches for are hidden behind strong noise that is produced by these complex, interconnected systems. With small data sets and/or strong noise, many different candidate models may describe the data to a similar degree. This model ambiguity often leads to overfitting as one tends to choose more complex models that fit the data a tiny bit better. However, one has to keep in mind that more simple models often generalize better to unseen data, so one has to find an objective trade-off between goodness-of-fit and model complexity (“Occam’s razor”).
The study of complex systems calls for novel methods that can “peak behind” the curtain of emergence, or at least not be mislead by it. While many researchers (and some practitioners) try to reduce the messy statistics of complex systems to ordinary Gaussian approximations by referencing the Central Limit Theorem, the lessons on pre-asymptotics by Nassim Taleb have shown us that such a shortcut does not work and can lead to substantial errors. Many still cling to old and established methods, despite new insights clearly exposing their weaknesses. For example, as Mark Spitznagel argues it in his book “Safe Haven”, reducing a portfolio to a set of expected returns and a covariance matrix will miss the most important effects that drive the interactions between financial assets – yet Modern Portfolio Theory does exactly this and is still widely employed.
At Artifact Research, we believe that Bayesian modeling, probabilistic programming, and model selection techniques make up a great set of tools to analyze complex systems, avoid overfitting, and solve problems involving scarce data, weak signals, and strong noise. We consult in using state-of-the-art tools, provide interactive educational resources, and offer innovative software products for the analysis of complex systems and small-data problems.
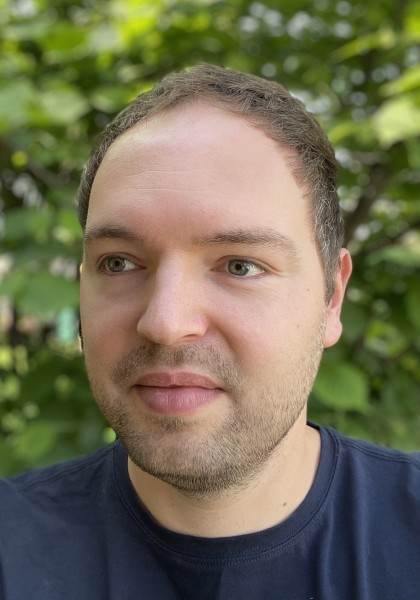
Dr. Christoph Mark
Founder & CEO
Trained physicist, likes probabilistic models and complex systems, from living cells to financial markets. Always searching for patterns in biological, financial, and business-related data. I founded Artifact Research to accelerate the transformation of ideas and projects related to complex systems into full-fledged products and worked-out insights.
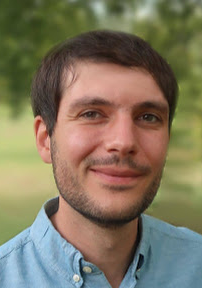
Dr. Richard Gerum
Founder & CEO
Trained physicist passionate about software development, with expertise in biophysics, machine learning, and neural networks. I focus on infrastructure, website development, user experience, and visualization at our company. After postdoc positions at the University of Erlangen-Nürnberg and York University, I co-founded this company to shape my own projects and take pride in seeing them used and appreciated. My recent work includes a Nature Physics publication, and you can learn more on my personal website or Google Scholar profile.